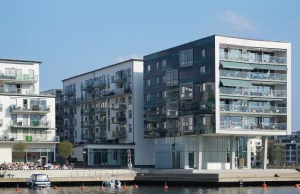
“In the DigiCityClimate project, we are trying to reduce citizens’ energy use with the help of AI. We will also help condominium associations balance and optimize their properties’ operation and make it easier for the City of Stockholm with energy advice,” says Anne Håkansson, researcher at KTH.
Today, condominium associations can turn to the Energy and Climate Advisory Service (EKR) at the Energy Agency for advice and support in reducing their energy use. The associations receive a report that shows, among other things, the property’s energy performance, the most significant ‘energy thieves,’ and a list of recommended energy-saving measures. This could involve upgrading existing 2-glazed windows to 3-glazed windows, adding additional insulation to the attic, replacing older washing machines, or installing solar cells.
“The workflow so far is time-consuming, and the energy advisors spend a lot of time collecting data about the building during site visits and in administrative systems. Instead, we want to use a conversational agent, a chatbot, which uses AI technology to produce the recommendations,” says Oleksii Pasichnyi, a researcher at KTH.
The data underlying the recommendations comes from several sources: Lantmäteriet’s building and property register, energy declarations from the Swedish National Board of Housing, Building and Planning, cultural classification and 3D models from the City of Stockholm, energy use from Stockholm Exergi, CO2 intensity from Svenska Kraftnät and Stockholms Exergi, and climate data from SMHI.
The aim is now to investigate how artificial intelligence can be used to produce recommendations for energy-saving measures.
Currently, a chatbot is linked to roughly 800 general questions and answers, see sparabot.com/
In the next step, the researchers want to make it possible to use context-dependent questions to generate answers, that is, to ask questions that are linked to a specific building. Large language models, such as OpenAI’s ChatGPT, are used to do that.
Eight condominium associations in Hammarby Sjöstad are part of a pilot project because the documentation of the properties’ energy performance and building data is extensive, including previously implemented energy efficiency measures. In addition to this, the external data sources mentioned above are included. When a query comes in from the condominium association, it is interpreted and processed by the language model, which has access to the data sources. A chatbot delivers energy efficiency measures with the best potential for the building’s owner.
“Why does our property get class F in the energy declaration, is an example of a question that can be asked and answered. The large language models are very good at retrieving and compiling this information,” says Oleksii Pasichnyi.
But can we trust the answers the models give? Shadaab Ghani, computer scientist at KTH, is also involved in the project. She says the language models used are expensive to develop, and they have already been trained. This means that they may be biased and based on corrupt data. It will, therefore, be essential to assess, test, and validate the proposed energy-saving measures before scaling up the AI support to users within the Energy and Climate Advisory Service.